FASHNAI
OOTDiffusion Review
OOTDiffusion is a new virtual try-on solution based on latent diffusion that was launched this month. It quickly gained popularity and became highly sought-after soon after its release. In this review, we will explore their capabilities and discuss any limitations.
Written by Aya Bochman | February 27, 2024
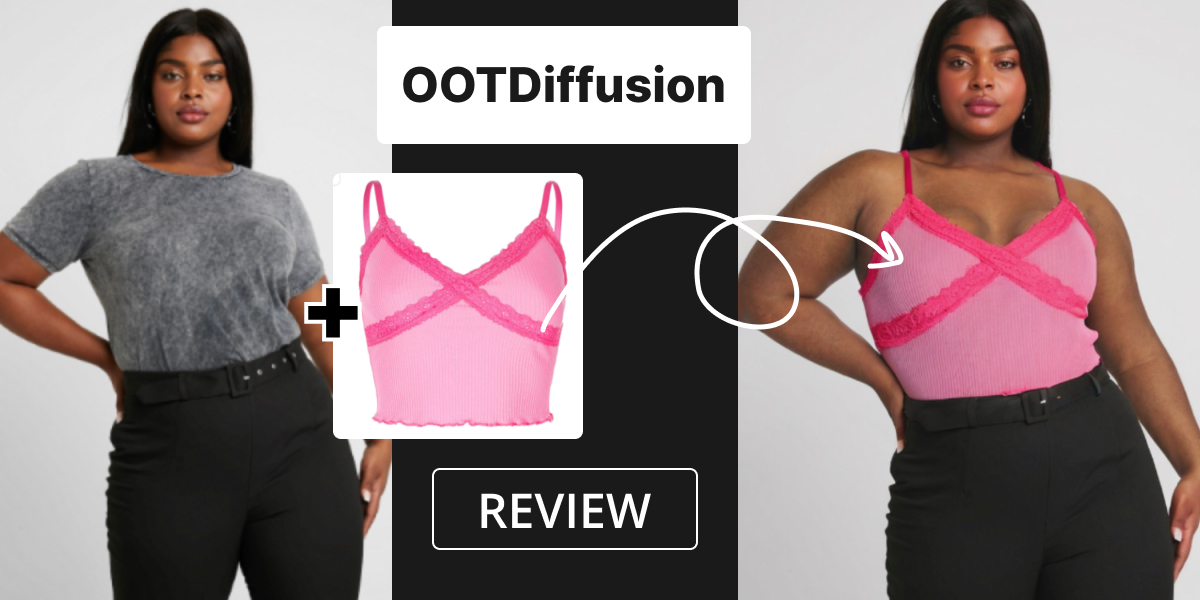
Overview
OOTDiffusion was introduced to us just this month by the research team from Xiao-i, a Shanghai based cognitive intelligence enterprise. They’ve published a virtual try-on demo for generating outfits on their example models, or a custom model of choice, and their achievements are quite noteworthy.
What's intriguing about them is that they're planning to release a paper soon, and their model architecture and inference code are open-source (Check our their GitHub repository). This has encouraged many enthusiasts in this domain to adopt it for their own use cases.
The demo permits the upload of a custom input model, but the garment image must be standalone, not worn on a model.
After extensive testing, we've gained insights into its strengths and potential, while also identifying some significant drawbacks that warrant attention.
In this experiment, we will explore generating results using:
A custom garment
A custom model
A Custom Garment
We won't display the result from a garment with a slightly detailed background, as opposed to a blank one, because the model struggles with this, resulting in a highly distorted outcome. However, with a garment image that has a clear background, the results can be truly stunning, as demonstrated in this example:
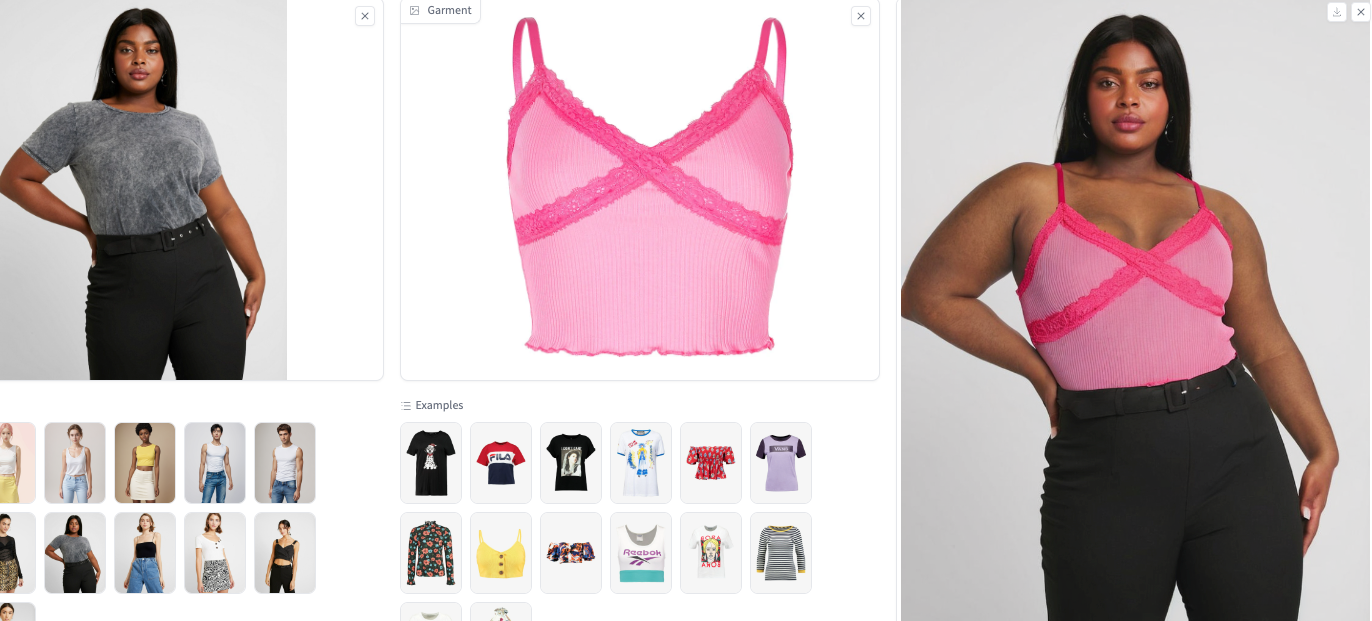
Notice the meticulous preservation of the model's details, skin color, and body shape. This result appears to be a ready-to-use creation.
We also experimented with generating a complex lower garment:
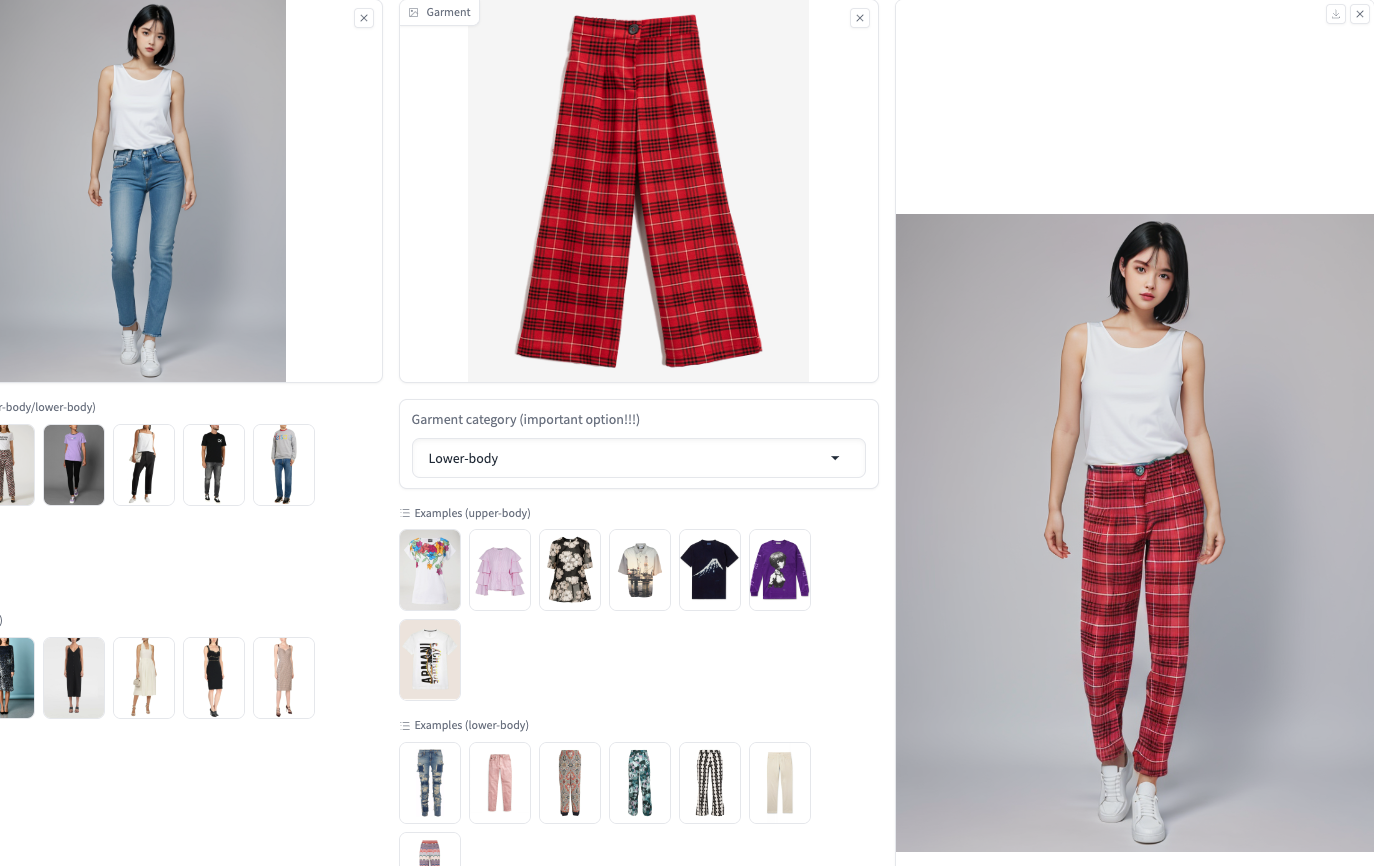
Once again, the details of the model appear to be perfectly preserved, and the overall result looks impressive. However, a minor issue we noticed is that the wide-shaped pants adopted the form of the input model's skinny jeans. This is a significant limitation we've observed with OOTDiffusion, which we'll further illustrate in the following example.
In the next example, we selected a complex patterned short strapless dress and used one of OOTD's suitable models for generating a dress. As evident in the result, the virtual try-on closely mimics the input model's garment shape.
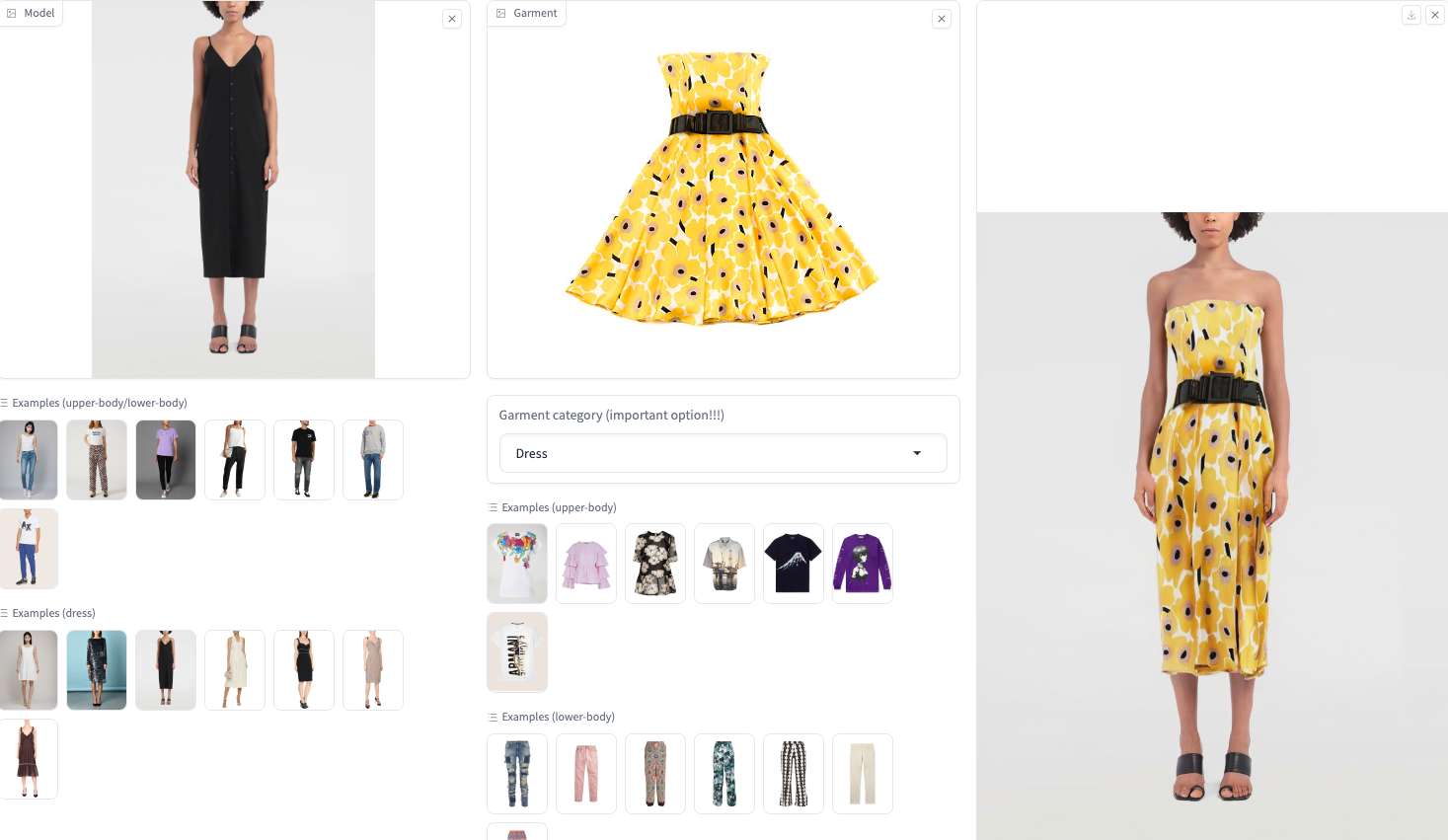
This means that generating a short dress on a model wearing a maxi dress is not feasible, and the same limitation applies to attempting to generate any type of garment on a model wearing a different kind (e.g., a skirt on bottoms, shorts on jeans, etc.).
The pattern, however, closely resembles the input garment and is executed remarkably well.
A Custom Model
While working with a custom model, we encountered two main issues. The first is the previously mentioned limitation: the model's inability to disregard the shape of the input model's garment.
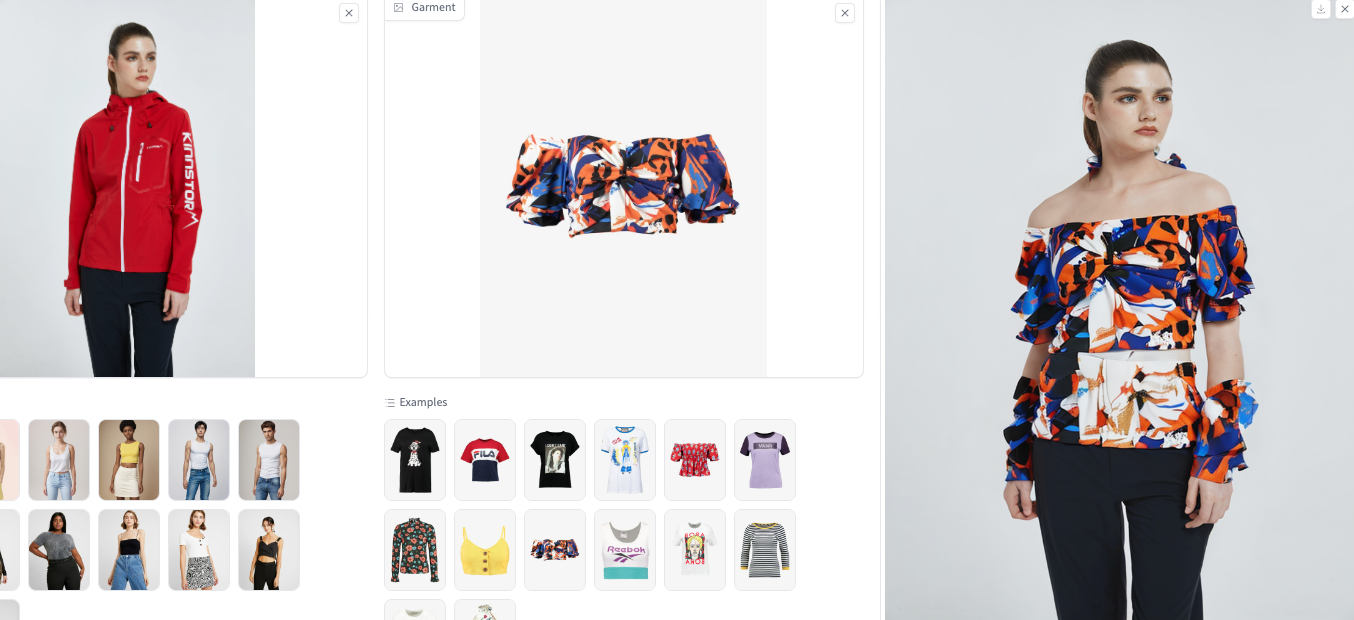
The second issue is the distortion of the model's background, which appears to be due to traces of mask artifacts, as clearly illustrated here:
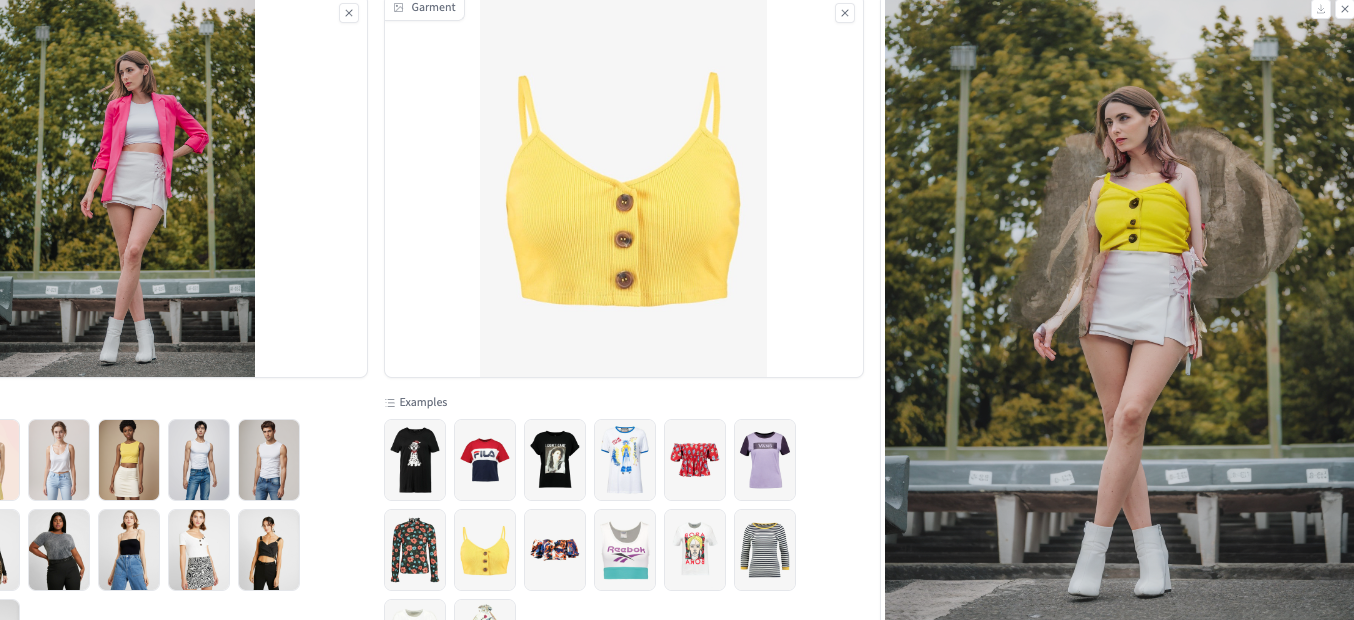
In both experiments, we selected garments from OOTD's examples to enhance the chances of a successful outcome, given that their model is specifically designed for them. However, the results did not meet our expectations.
In our final test, we used an input model with a blank background and tried to generate a similar type of garment (an upper body t-shirt). The results demonstrated an improvement:
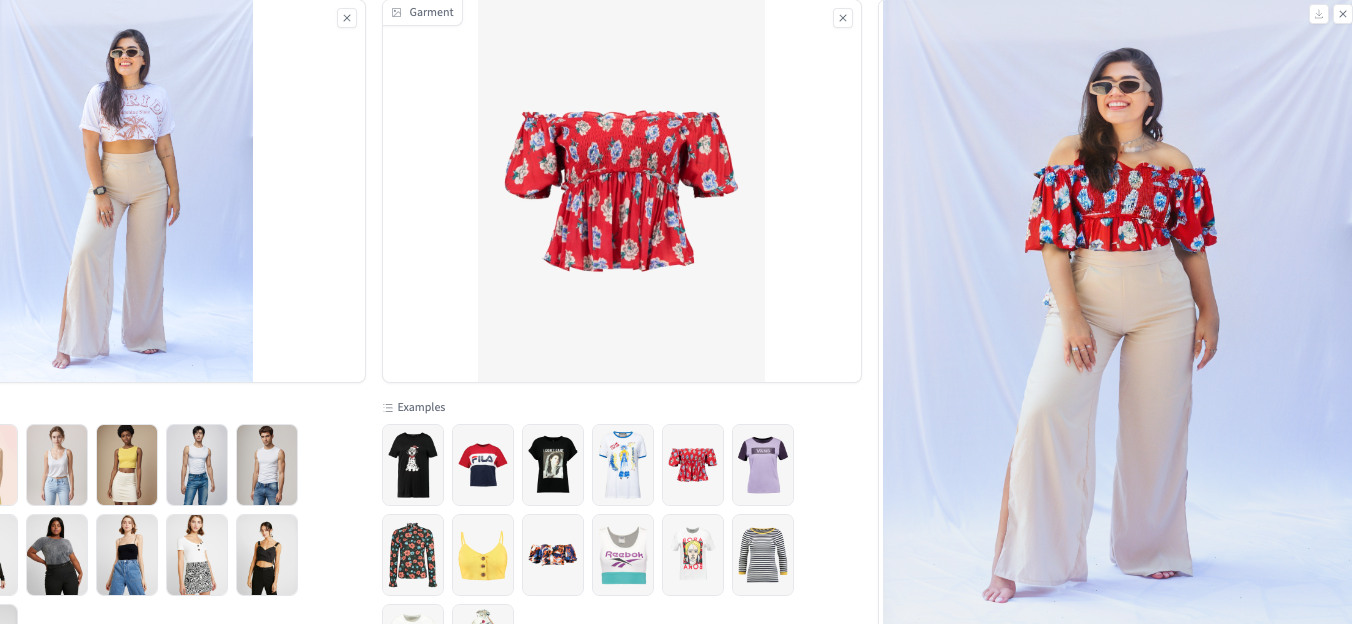
*Some of the results appear stretched because OOTDiffusion operates with a 768x1024 resolution, and our input image did not match this aspect ratio.
Conclusion
OOTDiffusion is certainly capable of producing high-quality results, provided you use clean images with blank backgrounds and ensure that the input model's garment is similar, at least in length, to the garment you intend to generate. We're excited to see how try-on solutions will evolve and eventually become mainstream in the fashion industry.